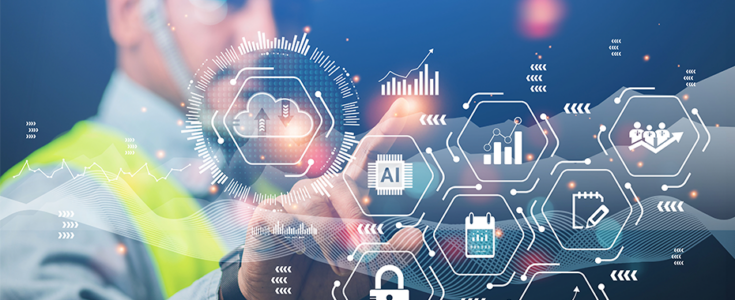
5 Use Cases of Predictive Analytics in Manufacturing
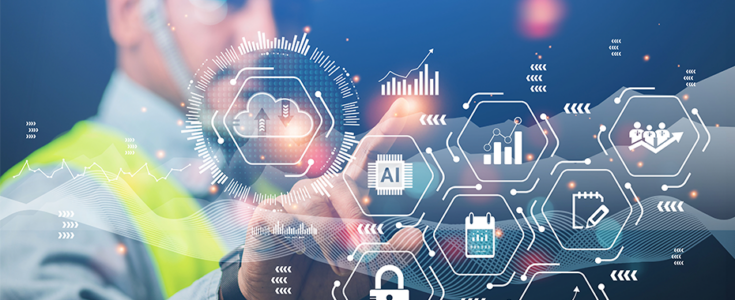
Increasingly, manufacturers are looking to improve their operational efficiencies by adopting new technologies. Predictive analytics is proving its worth in manufacturing. The market is projected to reach $2.5 billion by 2026, up from a market value of $535 million in 2018. Predictive analytics offer manufacturers greater insight into demand, risk, and quality. Historical data helps companies to identify patterns within the manufacturing process, improve strategic forecasts, and make better decisions. Companies can streamline processes, improve both product quality and production, and increase optimization, all leading to a positive bottom-line impact. Tasked with challenges relating to demand, cost, quality, and efficiency, manufacturers have implemented predictive analytics to not only address these challenges, but to position themselves for growth.
1. Demand Forecast
One of the top use cases for predictive analytics in manufacturing is demand forecasting. For manufacturers, the right data makes all the difference when trying to identify trends and gain a greater understanding of their performance. With this knowledge, they are better equipped to fulfill customer expectations and predict changes in the market. Materials, logistics, weather, and sales are just a few factors that need to be included in the equation for manufacturers to forecast demand.
Analytics enable manufacturers to make important calculations relating to production and sales, ultimately improving inventory management. With better inventory management, manufacturers can safeguard against over production and out of stock items, both of which can lead to revenue loss. With time and money factored in, for most organizations, predictive analytics can result in a positive ROI.
2. Improve Product Quality
Errors in the manufacturing process can lead to costly delays, product recalls, and dissatisfied customers. Unfortunately, all of these can tarnish an organization’s reputation, leading to a loss in customer trust and revenue. In the manufacturing process, there are still quality checks performed by humans; these manual operations open the door for oversights and errors. This is another instance in manufacturing where predictive analytics, artificial intelligence (AI), and machine learning (ML) can be implemented. With greater accuracy than humans, devices like cameras and sensors, controlled by computer models, can predict product defects. Early identification of these flaws helps organizations mitigate losses relating to production and recalls.
3. Inventory Management
As alluded to in the demand forecast section, inventory management is key to turning a profit. Using predictive analytics and computer models, supply chain managers can make better decisions when ordering, helping to improve accuracy, and resulting in better storage optimization. In addition to keeping shelves stocked and lessening the burden of dead stock, computer models can also assist supply chain managers with stock placement on shelves or in warehouses.
4. Improve Machine Performance/Maintenance
Machines are critical to the manufacturing process. Because breakdowns and repairs are so costly, manufacturers are increasingly using predictive analytics to help them take preventative measures to circumvent loss. In relation to machine performance and maintenance, data is powerful because it can help predict failure and automate maintenance. Routine maintenance schedules are essential because it prevents unnecessary downtime from occurring. AI can also keep your equipment running efficiently by preventing machine overuse.
5. Workforce Safety
In the manufacturing industry, workers are often exposed to safety hazards including heavy machinery, falls, hazardous materials, powered vehicles, and fires. Workplace safety is critical to preventing or minimizing injuries, illnesses, and, in some cases, even fatalities. When workers feel safe, that not only impacts employee morale, but output and production as well. Predictive analytics can play a key role in enhancing the safety of workers, which in turn, can be very beneficial to employees and the organization.
To ensure safety, organizations can use predictive analytics to help predict potential accidents. By taking proactive measures, accidents can be avoided. Further, AI can be used to automate dangerous and repetitive tasks that put employees at risk. In doing so, workplace accidents and manufacturing errors can be minimized.
Predicting Success in Manufacturing with Analytics
Manufacturers continually strive for cost-effective and less labor-intensive solutions to maximize output and quality. Any disruptions in the supply chain can drastically alter manufacturing processes, costing manufacturers time and money, while frustrating customers. Manufacturers are also racing to outperform their competition and meet customer demands while trying to attain their own profit expectations. To do this, manufacturers need to capitalize on every opportunity afforded to them.
Manufacturers generate large amounts of data, making them great candidates for predictive analytics. Predictive analytics enable manufacturers to make quality improvements, improve forecasting decisions, and boost employee morale and productivity by implementing safety procedures. Predictive analytics can also help businesses to avoid disruptions and capitalize on key opportunities. Thus, transforming their businesses, optimizing their strategies and setting themselves on a path to success.
Apexon understands that data is critical to any digital initiative. Without modern data and analytics capabilities, businesses operate at a competitive disadvantage to their industry counterparts. To learn more about predictive analytics, check out Apexon’s Data & Analytics services or get in touch with us directly using the form below.
FAQ’s – Manufacturing Analytics
Data analytics in the manufacturing industry refers to the process of collecting, analyzing, and interpreting large volumes of data generated throughout the manufacturing process to derive insights, optimize operations, and drive informed decision-making. It involves the use of various statistical and mathematical techniques to identify patterns, trends, and correlations within the data, ultimately aiming to improve efficiency, quality, and productivity in manufacturing operations.
In the manufacturing industry, several software tools are commonly used for data analytics, each offering unique features and capabilities tailored to different manufacturing needs. Some popular examples include:
- Tableau: A powerful data visualization tool that allows manufacturers to create interactive dashboards and reports to gain insights from their data.
- Microsoft Power BI: Another widely used business intelligence tool that enables manufacturers to analyze data, create visualizations, and share insights across the organization.
- MATLAB: A programming environment specifically designed for numerical computing and data analysis, often utilized for advanced analytics and modelling in manufacturing.
- Minitab: Statistical software commonly employed for quality improvement and statistical analysis in manufacturing processes.
These are just a few examples, and the choice of software often depends on factors such as the specific requirements of the manufacturing process, budget constraints, and the level of expertise within the organization.
A manufacturing data analytics dashboard typically includes a range of key performance indicators (KPIs), metrics, and visualizations that provide insights into various aspects of the manufacturing process. Some common components of such a dashboard may include:
- Real-time production metrics such as throughput, cycle time, and downtime.
- Quality indicators such as defect rates, scrap rates, and first-pass yield.
- Inventory levels and supply chain performance.
- Machine health and maintenance information.
- Energy consumption and environmental impact metrics.
- Overall equipment effectiveness (OEE) and other efficiency measures.
The dashboard is designed to provide a comprehensive overview of manufacturing operations, enabling stakeholders to monitor performance, identify trends, and make data-driven decisions to optimize processes and improve outcomes.
Several reputable courses are available for individuals seeking to enhance their skills in data analytics specifically tailored to the manufacturing industry. Some noteworthy options include:
- Coursera: Data Analytics for Lean Six Sigma: This course covers data analytics techniques applied within the context of Lean Six Sigma methodologies, focusing on process improvement and quality management in manufacturing.
- Udemy: Manufacturing Analytics using R Programming: An in-depth course that teaches participants how to leverage the R programming language for performing analytics tasks relevant to manufacturing processes, including predictive maintenance, quality control, and supply chain optimization.
- edX: Industrial IoT on Google Cloud Platform: This course explores the intersection of industrial Internet of Things (IoT) and data analytics, providing practical insights into how manufacturing organizations can leverage IoT data for analytics-driven decision-making using Google Cloud Platform services.
- LinkedIn Learning: Data Science and Analytics for Industry 4.0: A comprehensive course that delves into the application of data science and analytics techniques in the context of Industry 4.0, covering topics such as predictive maintenance, smart manufacturing, and predictive quality analytics.
These courses offer valuable knowledge and skills tailored to the specific challenges and opportunities present in the manufacturing sector, equipping learners with the tools they need to excel in data analytics roles within manufacturing organizations.