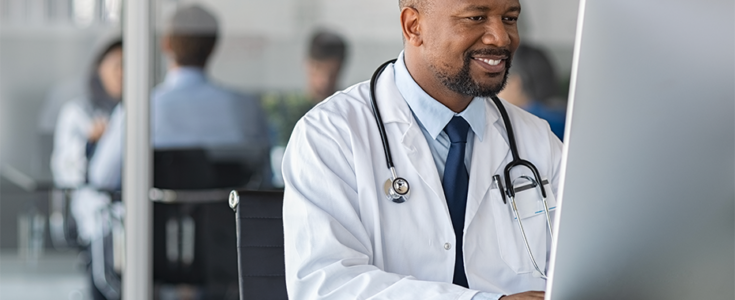
Why Improving Health Outcomes is Contingent on Understanding SDOH
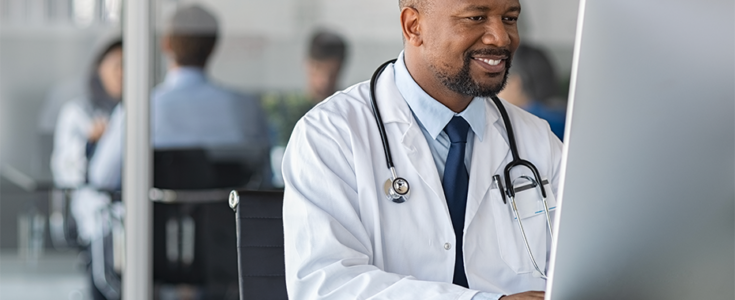
Non-medical factors like the condition in which people are born, raised, live, work, and age play a significant role in shaping a person’s overall health and health outcomes—just as much as their medical conditions. Numerous studies show that these non-medical factors, also known as social determinants of health (SDOH), account for 30-55% of health outcomes (WHO). SDOH have a major impact on people’s health, well-being, and quality of life. In the USA, there is a 15-year discrepancy in the life expectancy of the richest and poorest men (10 years for women).
The Factors Affecting SDOH
SDOH are characterized by geographic conditions and health behaviors that are influenced by historical and social factors.
Examples of SDOH include:
- Safe housing, transportation, and neighborhoods
- Racism, discrimination, and violence
- Education, job opportunities, and income
- Access to nutritious food and opportunities for physical activity
- Polluted air and water
- Language and literacy levels
These social determinants contribute to persistent chronic disease disparities among racial, ethnic, and socioeconomic groups in the United States, systematically limiting opportunities for marginalized populations to be healthy. Increasingly, organizations are turning to big data, analytics, and AI to better understand and respond to health inequity.
The Challenges in Addressing SDOH
The issues surrounding SDOH are broad-ranging and complex, and tackling its inequalities requires policy, systemic, environmental, and programmatic changes. While we understand the generic factors influencing health outcomes, applying these in communities with the worst health outcomes has been challenging.
SDOH are particularly difficult to address in rural communities thanks to resource constraints, cultural factors, and a lack of awareness of how these factors affect health. Understanding rural cultural values can help to facilitate successful programs that tackle SDOH. For example, some rural programs that focus on SDOH have sought to connect residents to social services such as food, housing, and transportation assistance. Rural residents may feel concerned and unwilling to make full use of the services because of the stigma surrounding access to certain types of medical services (like mental health) in a community setting where anonymity is impossible.
Community health assessments and long-term planning are required to improve health inequities. But without the added context that advanced analytics brings, these measures are not enough. Health organizations are now beginning to harness data analytics from a wide range of sources, couple it with their own patient data, and use the insight to guide targeted changes that improve outcomes.
The Role of Big Data, AI, and Analytics in Improving Health Outcomes
One of the most significant breakthroughs to emerge in the quest for health equality are solutions that use AI and ML to further our understanding of the impacts of SDOH and shed light on local opportunities to address specific community challenges. Previously, analytics into SDOH were conducted using generic data over large populations or using surveys that could easily provide skewed results. Using data transformation techniques and AI/ML modeling, public bodies and health organizations can now drill down into the specific local factors affecting health equity and fix them.
In order to gain a holistic understanding of medical and non-medical factors influencing health outcomes in a community, healthcare organizations need to ensure their data platforms can integrate third-party data sets – such as those relating to SDOH – with their existing, anonymized patient data to analyze the issues impacting healthcare provision. Currently available SDOH data examples include socioeconomic status, employment, and healthcare access. Other closely related data sets include patient-reported outcomes data that can be used in barrier identification, market segmentation, and therapeutic journey analysis to advance equity in trial and care delivery and improve the health of populations.
Easy Data Integration to Unlock 360-Degree Insights
A service that is tailor-made for customers who need to find, subscribe to, and use third-party data in the cloud is AWS Data Exchange. It is the only data marketplace with more than 3,500 products from over 300 providers delivered – through files, APIs, or Amazon RedShift queries – directly to the data lakes, applications, analytics, and machine learning models that use it.
Using AWS Data Exchange, healthcare organizations can streamline all third-party data consumption from existing subscriptions —which healthcare companies can migrate at no additional cost to themselves or the provider— to future data subscriptions, in one place. AWS Data Exchange is secure and compliant. It is fully integrated with other AWS data analytics services and third-party tools and offers consolidated billing and subscription management.
Powerful Analysis That Drives Health Improvements and Efficiencies
The ability to use and share this data is a prerequisite for analytics. In health and life sciences, where the data often carries sensitive information, organizations must be able to leverage it in such a way that complies with important security, privacy, and industry regulation. Solutions like the Patient 360 healthcare framework powered by Apexon’s Healthcare Data Analytics Platform combine anonymizing techniques with powerful analysis to consolidate data across the healthcare delivery system. It is designed to provide a unified view of the patient, predicting risk factors and correlations between different medical and non-medical determinants.
Patient 360 powered by HDAP is built on event-driven micro-services and serverless technologies on AWS which ensures healthcare organizations benefit from the scalability, security, reliability, and cost-effectiveness of the cloud without compromising performance.
At the core of HDAP’s value proposition are its transformational advanced analytics and machine learning capabilities which healthcare organizations leverage to build, train, and deploy powerful machine learning models.
- Integration of different types of data for multiple uses and users across the healthcare organization
- Billing data for administrators
- Patient diagnostics and treatments for physicians
- Adverse conditions/reactions for nurses
- Data analysis for researchers
- Capturing and sharing patient medication histories with other providers and public health organizations to ensure safer and more effective care in compliance with HIPAA regulations
- Mapping and validation of critical patient care codes to ensure accurate and complete information is available for accurate patient care, tracking, and billing
- Data tagging to provide added context and checkpoints to existing data for better data visibility, verification, and application
- Standardizing healthcare classification codes [ICD, SNOMED], procedure codes [CPT], labs results code [LOINC], medication and drug codes [NDC], image-related codes [DICOM] and more to enable more intuitive care analysis, correlation, and comparative research with other countries
- Easy analysis and insight following the mining of clinical notes in unstructured formats like free text
- Mapping and optimizing core data that might use different labels in different environments to make it more consumable, searchable, and actionable.
A 360-Degree Approach to Transforming Health Outcomes
Social determinants of health influence the health outcomes of individuals and communities as much as, if not more than, medical factors. While the global community has understood this for many years, progress toward health equity has been slow. Advances in cloud processing, big data analytics, and AI/ML now make it possible for health and life sciences organizations to connect the dots between medical and non-medical health factors influencing patient outcomes.
AWS Life Sciences Competency partner Apexon is a digital-first technology services firm that works closely with healthcare and life sciences organizations to deliver human-centric business transformation. Apexon leverages data solutions based on the AWS platform to help health companies understand health outcomes in the widest possible context.
Apexon and AWS have partnered to create a powerful set of tools that healthcare organizations can use to harness disparate data and create an all-round view of the patient. Furthermore, solutions like Patient 360 powered by Apexon’s HDAP enable organizations to focus on providing insights and accelerating transformational change in the areas that need it most. Together, Apexon and AWS are enabling healthcare organizations to take radical, data-driven steps to improve health equity and efficiency. For more information on how Apexon is humanizing patient care with HDAP – powered by Patient 360, take a look at this fact sheet.
This blog was cowritten by Mike Boese, Vice President of Data Science at Apexon and Rahul Ghate, Head of Worldwide Partner Development for Healthcare and Life Sciences Data Analytics at AWS. This topic was originally discussed in an HIMSSCast podcast episode titled “AWS and Apexon present: Unlock full potential of SDOH and improve patient outcomes with Patient 360 and Artificial Intelligence.”