Digital pathology is transforming healthcare through advanced imaging, artificial intelligence, and data-driven technologies. This comprehensive article explores how cutting-edge digital pathology solutions are reshaping diagnostic accuracy, research capabilities, and patient outcomes across global healthcare systems. Process flows, technical points, implementation plans, and real-world examples show how data interoperability can help. This technology can change how healthcare is delivered.
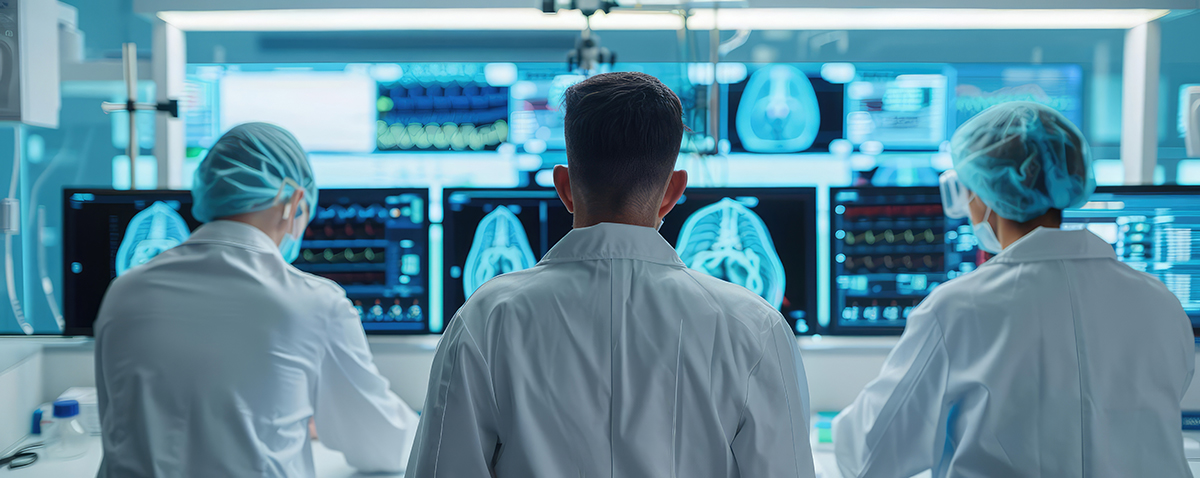
Introduction
Pathology has long been the cornerstone of medical diagnostics, offering vital insights into disease mechanisms, patient management, and therapeutic planning. Traditional pathology looks at glass slides under a microscope. This method has been very helpful, but it faces challenges from the more complex needs of modern healthcare systems. This analogue approach presents significant limitations, such as, restricted accessibility, the time-intensive nature of manual analysis, risks of sample degradation, and barriers to efficient data sharing.
Also Read: Two Use Cases of Digital Twins in Healthcare
Digital pathology addresses these challenges by converting glass slides into high-resolution digital images, enabling streamlined workflows, enhanced collaboration, and integration with computational tools. In an era where personalized medicine, digital diagnostics, and data-driven research are key, digital pathology is becoming a crucial technology. It is changing the way healthcare systems handle diagnostics, research, and education. By integrating advanced imaging with artificial intelligence (AI), this technology is paving the way for more accurate, efficient, and scalable pathology workflows.
Benefits of Digital Pathology
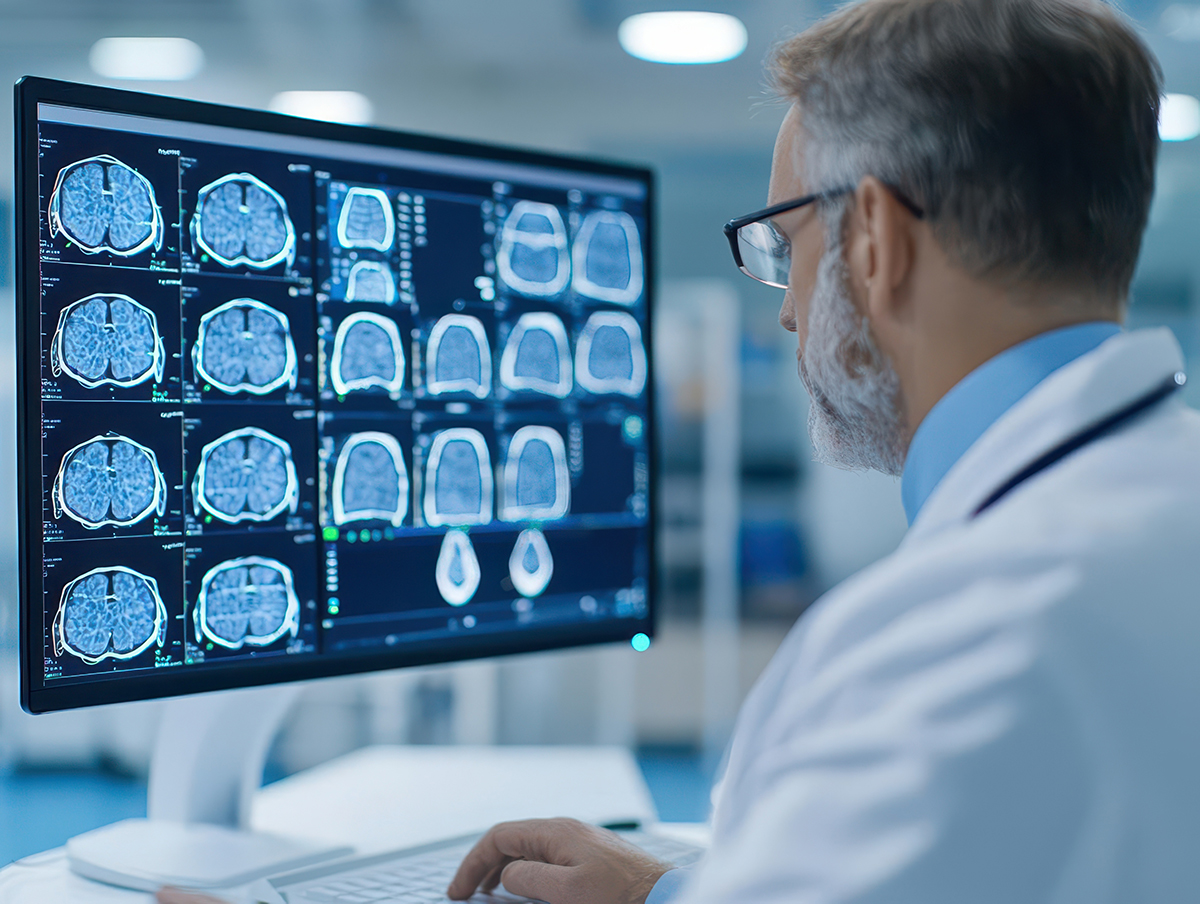
Enhanced Diagnostic Accuracy
- AI in Pathology: Artificial intelligence and machine learning algorithms are revolutionizing the field by providing diagnostic support in complex cases such as oncology, infectious diseases, and neurodegenerative conditions. These algorithms analyze vast amounts of imaging data, identifying subtle patterns, and quantifying features that may not be discernible to the human eye. For example, AI models can predict tumour grades, identify molecular markers, and even assess treatment response.
- Error Reduction: By standardizing image analysis and reducing reliance on manual interpretation, digital pathology minimizes diagnostic errors. Automated tools ensure consistent application of diagnostic criteria, reducing interobserver variability.
- Remote Consultations: Digital platforms enable seamless sharing of slides between pathologists, clinicians, and researchers across the globe. This facilitates real-time second opinions, interdisciplinary consultations, and collaborative decision-making, ultimately improving patient outcomes.
Also Read: Fine-Tuning AI as a Catalyst for Medical Innovation
Improved Workflow Efficiency
- Faster Turnaround Times: Automated slide scanning and computational analysis reduce the time required for diagnostics. This is particularly beneficial in high-throughput settings such as cancer centres or emergency departments, where rapid diagnoses can significantly impact treatment outcomes.
- Centralized Digital Archives: High-resolution digital archives eliminate the need for physical slide storage, reducing risks of loss or damage. These archives integrate with laboratory information systems (LIS) and electronic health records (EHRs), enabling instant retrieval of patient data.
- Streamlined Processes: Automated workflows, including image pre-processing, staining quality checks, and diagnostic flagging, save time and labor. Pathologists can focus on complex cases rather than routine analysis.
Integration with Research
- Big Data Utilization: The digitization of pathology slides creates extensive datasets that can be used for AI training, multi-center studies, and advanced statistical analyses. These datasets provide a foundation for discovering new diagnostic and therapeutic pathways.
- Biomarker Discovery and Validation: Digital imaging enables detailed analysis of tissue architecture and molecular characteristics, facilitating the identification of novel biomarkers. These discoveries are critical for developing targeted therapies and advancing precision medicine.
- Clinical Trials and Retrospective Studies: Digital archives allow researchers to revisit historical cases for hypothesis generation, validating new diagnostic techniques, or conducting longitudinal studies.
Also Read: Top 3 Use Cases of AI From a Healthcare Technology Leader
Educational Advancements
- Virtual Microscopy and Training: Digital slides enable virtual microscopy, allowing medical students, trainees, and researchers to study and annotate samples from any location. This fosters global knowledge exchange and makes pathology education more accessible.
- Standardized Learning Materials: Digital platforms provide consistent teaching materials, ensuring that all trainees have access to the same high-quality images and annotations. AI-powered platforms can also assess trainee performance, offering personalized feedback.
- Simulated Environments: Advanced simulation tools recreate real-world diagnostic scenarios, enabling trainees to hone their skills in a risk-free environment.
Process Flow in Digital Pathology
- Slide Preparation Biological specimens undergo a series of standardized histopathology processes, including fixation, embedding, sectioning, and staining. Automation in staining and sectioning ensures reproducibility and high-quality results, which are critical for digital scanning. This stage often involves optimizing staining protocols to highlight specific cellular or molecular features, ensuring consistent diagnostic outcomes.
- Slide Scanning Whole-slide imaging (WSI) devices digitize prepared slides by capturing high-resolution images with true-to-life color fidelity. Advanced WSI systems offer multi-plane z-stack imaging, enabling 3D visualization of tissue sections. Scanning parameters such as resolution, magnification, and lighting conditions are optimized for specific diagnostic needs. These devices also feature real-time quality control mechanisms, ensuring that every scan meets diagnostic standards.
- Image Storage Scanned images are securely stored in centralized or cloud-based databases. Storage systems are integrated with metadata, including patient demographics, staining protocols, and diagnostic notes. Cloud platforms provide scalability and accessibility, ensuring uninterrupted access to images. Advanced encryption methods safeguard data, ensuring compliance with privacy regulations such as HIPAA and GDPR.
- Image Analysis AI-powered software analyzes digital slides, detecting patterns, classifying abnormalities, and quantifying features such as nuclear size, cellular morphology, and proliferation indices. These tools generate heatmaps, annotations, and automated measurements that assist pathologists in making precise diagnoses. Additionally, advanced algorithms can predict disease progression, enabling proactive therapeutic interventions.
- Report Generation Automated reporting tools compile diagnostic data into comprehensive reports, including annotated images, measurements, and AI-derived insights. Pathologists review and validate these reports to ensure clinical accuracy before sharing them with treating physicians. Reports are often customized to align with specific institutional or regulatory guidelines, ensuring usability across diverse clinical settings.
- Data Sharing and Collaboration Interoperable platforms allow seamless sharing of digital slides and diagnostic reports with multidisciplinary teams, external consultants, and research collaborators. Encrypted communication channels and access control mechanisms ensure data security and compliance with regulations. Collaborative tools enable real-time discussions, accelerating consensus-building for complex cases.
Data Interoperability in Digital Pathology
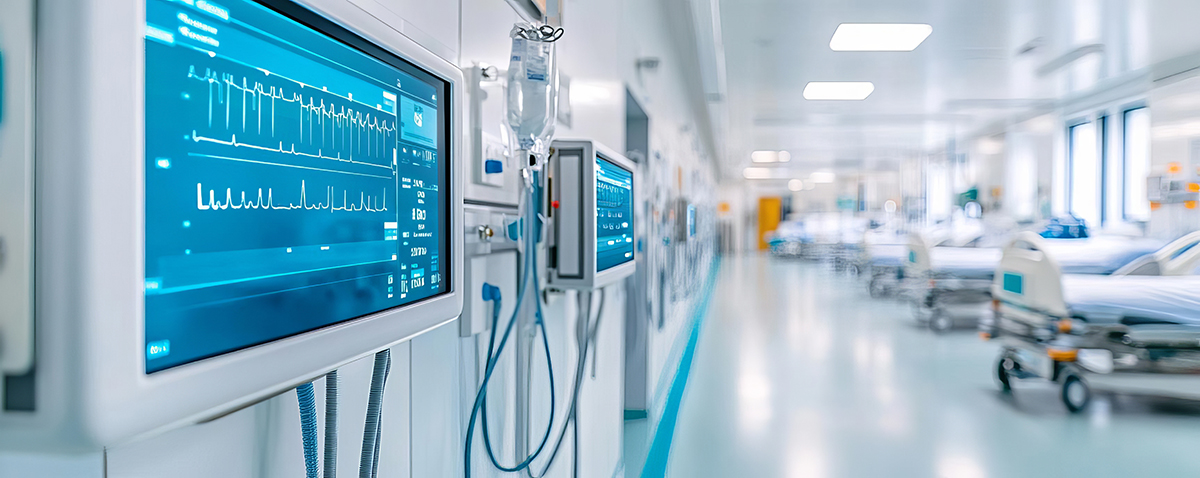
Importance of Interoperability
- Integration with Healthcare Technology and Systems: Interoperability enables digital pathology systems to seamlessly interact with EHRs, LIS, and radiology systems, creating a unified patient care ecosystem. This integration streamlines clinical workflows, reduces administrative burdens, and enhances decision-making.
- Regulatory Compliance: Compliance with global privacy regulations such as HIPAA and GDPR is facilitated through interoperable systems that manage data access and sharing responsibly.
- Cross-Institutional Research: Interoperable platforms foster collaboration by allowing secure sharing of anonymized datasets across institutions, accelerating research efforts. This is particularly crucial in multi-center studies and international collaborations.
Standards and Protocols
- DICOM for Pathology: The extension of DICOM standards to digital pathology ensures uniformity and compatibility across imaging platforms. DICOM facilitates integration with radiology and other medical imaging modalities, creating a cohesive diagnostic environment.
- HL7/FHIR Integration: These protocols streamline data exchange between digital pathology systems and other healthcare IT infrastructures, enhancing workflow efficiency and data accessibility. Advanced APIs ensure seamless interoperability, reducing integration challenges.
Case Studies
- Enhancing Oncology Diagnostics A leading cancer center implemented an end-to-end digital pathology solution, integrating WSI with AI-powered diagnostic algorithms. This initiative reduced diagnostic errors by 20% and improved turnaround times by 30%. Digital archives facilitated retrospective analysis, driving innovations in cancer treatment strategies. Additionally, the platform supported clinical trials by providing real-time access to annotated datasets.
- Accelerating Biomarker Discovery A global consortium of research institutions adopted a unified digital pathology platform, enabling the sharing of large, annotated datasets. This collaboration led to the discovery of a novel biomarker for early-stage Alzheimer’s disease, accelerating the development of diagnostic tests and therapeutic interventions. By leveraging AI-driven analytics, researchers achieved unprecedented insights into disease mechanisms.
- Expanding Access to Rural Healthcare A telepathology initiative in underserved rural areas enabled local clinics to digitize and transmit pathology slides to urban medical centers for expert analysis. This approach significantly improved diagnostic accuracy and reduced time-to-treatment, demonstrating the potential of digital pathology to bridge healthcare disparities. Mobile scanning units further extended this capability, ensuring access in the most remote locations.
Challenges
- High Initial Costs: Implementing digital pathology requires substantial investment in infrastructure, equipment, and training. Cost-effective strategies and government subsidies can mitigate these barriers.
- Data Management: High-resolution images generate enormous datasets, necessitating robust storage solutions and scalable computational infrastructure. Cloud-based solutions and edge computing are emerging as potential strategies to address these challenges.
- Resistance to Change: Transitioning to digital workflows requires cultural and operational shifts, often met with resistance from pathologists accustomed to traditional methods. Comprehensive training programs and user-friendly interfaces can ease this transition.
Future Directions
- AI and Deep Learning: Emerging AI technologies promise to enhance diagnostic precision, predictive analytics, and workflow automation. Integration of multi-modal data, including genomic and proteomic information, will further expand diagnostic capabilities.
- Global Standards: Universal standards for digital pathology interoperability will drive widespread adoption and foster cross-border collaborations. Initiatives by organizations like the DICOM Standards Committee and HL7 are paving the way for standardized implementation.
- Telepathology Expansion: Scaling telepathology solutions can democratize access to diagnostics, particularly in low-resource settings. Portable WSI devices and satellite-based communication networks are critical enablers of this expansion.
- Regulatory Frameworks: Evolving regulations will support ethical data sharing, fostering innovations in clinical and research applications. Collaboration between industry stakeholders and regulatory bodies will ensure balanced frameworks.
Conclusion
Digital pathology represents a paradigm shift in medical diagnostics, research, and education. By integrating advanced imaging, AI, and robust data management, this technology enhances diagnostic accuracy, accelerates research, and expands access to quality healthcare. As digital pathology continues to evolve, its potential to transform healthcare delivery and research will grow, paving the way for a future defined by precision medicine and global collaboration.
Also read: Understanding Gibberlink Mode: AI’s Next Leap – How Do We Ensure It Stays in Check?
Also read: AI in Denial Management – A Smarter Approach to Claims