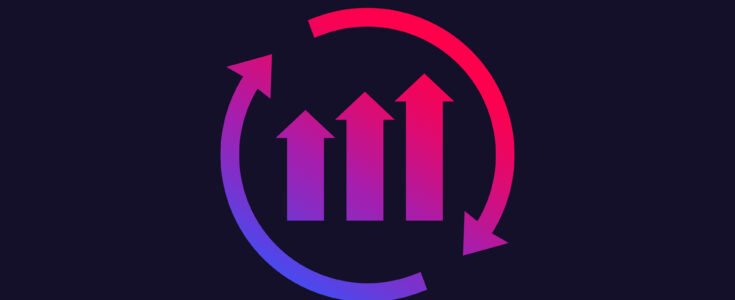
5 Success Factors for AI Projects
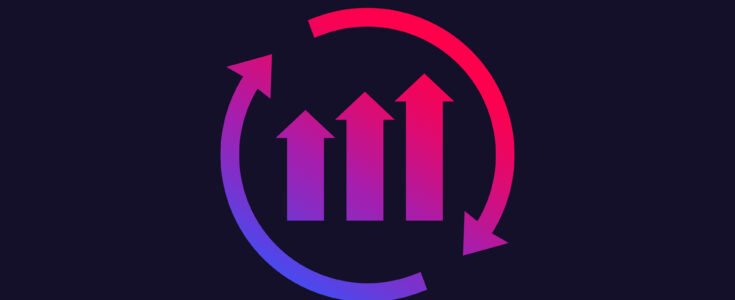
AI solutions have the power to change the way business operate. Applied intelligently, the technology can reinvent business processes, create better user experiences, and augment and enhance human decision-making. Little wonder that it’s seen as one of the biggest areas of opportunity for businesses today – and a core component of most digital transformation efforts. AI feeds off data, and for the majority of enterprises which are experiencing data overload, this can present a problem. Firstly, the “garbage in, garbage out” principle means that your data needs to be in good shape to deliver meaningful results. Secondly, data comes in all shapes and sizes and most of it is unused. This untapped resource is commonly known as “dark data”. A recent study of UK enterprises estimates that over half the data in enterprises can be classified as dark, nearly 30 percent is “redundant, obsolete or trivial” (ROT) and only around a fifth is business critical data.
When it comes to AI projects, data preparation is a critical task, and one that often trips up even the most conscientious task force. The challenge stems from the ever-growing quantity and the sheer range of data types, leaving enterprises drowning in a state of data paralysis. Despite AI and data analytics remaining game-changing priorities according to Gartner’s CIO survey, the majority of AI projects (still!) do not deliver. What’s behind this high failure rate? Factors range from inflated and inaccurate expectations to underlying technology challenges to resourcing. However, keeping AI initiatives on course shouldn’t be seen as daunting, so long as enterprises keep in mind the main success criteria.
- Prioritize problem areas
When it comes to AI, there’s a tendency to bite off more than you can chew. I’m convinced this is at least in part because most of the messaging around AI adoption emphasizes how valuable it is to gather data from all across the business. This is true, and it’s one of the foundational principles behind Apexon’s unique COMPASS methodology around continuous data intelligence, but it is also true that you can’t run before you can walk. It can become all too easy for enterprises to get very busy dealing with, categorizing and cleaning data with no end in sight and no real progress towards strategic goals being achieved. The answer lies in prioritizing the areas where AI adoption will deliver the most value. Such pilot schemes are far more likely to result in success because their scope is clearly defined. In our experience, providing teams with a project leaderboard through the AI technology service platform is a simple yet effective way to condense the complex work taking place in parallel streams and show progress and productivity. We use gamification techniques to keep teams engaged and motivated.
- Take an intelligence-driven approach to data preparation
Getting a mound of data “ready” for AI to perform its magic can turn into an arduous and potentially endless task. When people in the industry complain about “data paralysis”, this is the stage they are talking about. This state of paralysis can be mitigated by having a clearly defined scope and goal for the AI initiative, as described previously. However, the approach we have found to be most useful is to use accelerators to automate data prep as much as possible while simultaneously focusing on accessing data intelligence from existing tools in the SDLC on an ongoing basis.
Rather than diving into their unstructured data with little indication of how valuable it will end up being, teams benefit from getting actionable results earlier, which allows them to decide how to refine and improve the system as they move forward. Practically speaking, prepping data can be time-intensive and resource allocation is an important consideration. By gaining continuous insight from existing processes, enterprises can tweak their algorithms to significantly improve efficiency and quality. It allows enables teams to stay focused on the outcomes they’re trying to achieve.
- Use the right skills at the right time to maintain motivation and momentum
Deploying the right resources at the right time is an important success factor for AI adoption. Keeping projects on track requires enterprises to dynamically allocate skilled team members to maximize efficiency, maintain progress and keep morale up. Many will choose to work with external experts. This can be particularly valuable to ensure momentum, and it helps overcome skills gaps that would prevent an enterprise from taking on the project internally. Apexon has a number of digital accelerators specifically designed to help enterprises automate and speed up the “heavy lifting” associated with AI adoption. As well as partnering with external digital engineering experts, it’s also important for enterprises to tap into the expertise within their organization. Working closely with the subject matter experts who’ll be using your data-driven intelligence on a daily basis is one of the ways to assure that the data your AI project delivers is valuable from the start.
- AI needs to be agile
The best AI systems are not static. Remaining responsive is one of the benefits of following Agile methodologies, and this applies to AI adoption as well as to other areas of the DevOps cycle. Smaller groups working on focused projects, where team members can work in small cycles and iterate often are far more likely to progress than when enterprises engage in a company-wide AI initiative. This is true of all kinds of change management and digital transformation initiatives, but especially in cases where AI is automating or augmenting current processes. Employees can be cautious or concerned about AI before they understand how it can help them. People find it much easier to get behind an AI project once they see evidence of it working well as a pilot project within the organizations.
- Using intelligence to evolve
So far, I’ve emphasized the importance of small teams, prioritizing projects, short cycles, smart data prep and optimizing the mix of skills and technology available. These factors help maintain momentum while avoiding over-ambition and data paralysis. When it comes to maximizing the value of the insights being derived, while at the same time improving efficiency and reducing risk, the best way to achieve this is system of continuous intelligence. Such systems, like Apexon’s COMPASS platform, create a continuous feedback look in which the AI platform collects data from existing project management tools like JIRA and software value chains used for DevOps and enables real-time insights that teams can learn from immediately, and improve as they go. Keeping AI projects on track is about ensuring teams don’t lose sight of goals. A system of continuous innovation with intelligence at its core helps teams stay focused on outcomes rather than output, use data as a strategic decision-making asset and maintain progress towards KPIs whether they are business, process, technology or people-centered.
You can find out more about Apexon’s COMPASS approach to continuous intelligence that helps enterprises embed data-driven insights into the core of what they achieve. You can learn more about it in this webcast. Or, to talk to us about your specific AI challenge, fill in the form below.
Also read: Cost Optimisation for AWS SageMaker in GenAI Real-Time Inference Endpoints