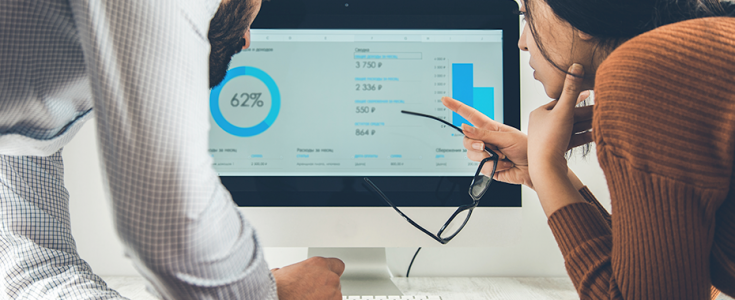
7 Data Quality Best Practices to Boost Enterprise Decision-Making
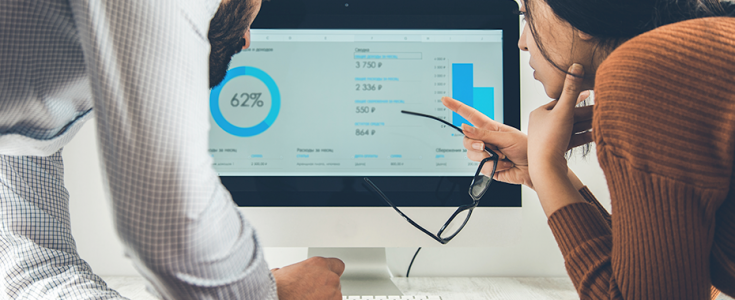
The adage, “trash in, trash out” is often used to summarize the need for quality data inputs to get reliable, valuable outputs. It is pointless to expect an algorithm to deliver worthwhile insights if it is fed with low quality or incorrect data. When an algorithm obviously delivers bogus answers, businesses clearly see the need to improve data quality. However, the impacts of bad data aren’t always so glaringly obvious.
In fact, it’s all too easy for organizations to live with data that’s duplicated, incomplete, or in disparate states and locations. The impacts might be felt as minor conveniences and frustrations, laggy systems, a reliance on guesswork, or inefficiencies due to manual processes. Worse still, low-quality data has a direct impact on customer engagement and lead generation.
The Hidden Cost of Bad Data
Poor quality data costs enterprises on average $12.9 million per year, according to Gartner. For an issue that’s inherently fixable, that’s a staggering loss to endure. Moreover, industry studies have estimated low-grade data costs the US economy about three trillion dollars every year, while the UK government says businesses spend between 10-30% of revenue on handling data quality issues.
While the financial impact is clearly significant, it may only be the tip of the iceberg. Low data quality percolates right through an enterprise with repercussions ranging from reduced revenues, decreased productivity, operational inefficiency, missed business opportunities, and increased exposure to regulatory, security, and compliance risks.
Furthermore, data quality issues aren’t going away any time soon: Gartner estimates that one third of businesses are investing heavily ($1 million or higher) in data-reliant initiatives that enable business agility and provide competitive edge. As enterprises rapidly digitalize their systems and ramp up investments in big data, analytics, and AI, they need to be assured of the quality of their data.
Challenges in Tackling Data Quality
The sheer volume of data often means that efforts to tame it end up overwhelming enterprises. Working to transform data – whether at the ingestion, ETL, or pipeline creation phase – can be time-consuming work, especially if the project scope is wide-ranging.
Big data projects can quickly lead to mounting costs and missed project timelines if they’re not carefully managed. This is partly because data pros are in such high demand that they’re an over-stretched resource and partly because it’s easy for seemingly more urgent projects to take priority.
All these factors point to the need for data quality management to be seen as part of a wider system of governance. Not only does good data governance improve agility and decision-making potential, it’s also the most reliable way of ensuring adherence to privacy rules and other regulations.
The specific steps a company needs to take to improve data quality will vary depending on many factors including overall digital maturity. However, there are best practices all companies should follow when addressing the challenge of data quality.
- Make data quality everyone’s priority
Data quality isn’t an abstract debate between data scientists, but rather a real issue impacting everyone in an enterprise. Whether they’re in sales, marketing, customer service, legal, finance, or HR, data quality is everyone’s responsibility and so a cross-functional approach is requited. This starts with senior level buy-in capable of delivering change across business units. Next, educate employees to understand how the data they touch is used. Finally, highlight easy actions employees can take to better gather and understand data.
- Embark on a data quality overhaul
A targeted data quality project can be a valuable intervention if data management practices are out-dated or if data quality is compromising agility or decision-making. Typically, this will involve data standardization, deduplication, matching, reporting, and profiling – all common challenges that lead to “dirty data.”
- Embed data quality standards into the data governance
While this kind of overhaul can provide a much-needed boost to data practices, a long-term approach is also required – this is where data governance can help. A data governance strategy will first answer the essential question of what constitutes acceptable data quality for a particular organization’s needs. Faced with a huge volume of various data types from internal and external sources, a comprehensive data governance program considers context, requirements, and business value. It will also keep enterprises accountable when it comes to maintaining accurate, consistent, timely data that conforms to all necessary regulations.
- Leverage AI/ML, analytics, and automation
Because big data is, well, so big, enterprises need a helping hand organizing the growing volumes of data that surround them. This boost comes in the form of automation. Bots are used to perform many of the tasks associated with cleaning up data: optimizing, de-duping, cataloguing, metadata handling, to name a few. These tools dramatically reduce data transformation times to help ensure rapid ROI on projects.
- Choose the right tool for the job
There’s no shortage of good data quality and management solutions, but this can make it hard to know which ones will integrate best with existing systems or future requirements. As you might expect, a digital engineering specialist like Apexon has extensive experience working with the leading cloud and data analytics providers, helping enterprises take full advantage of platforms like Azure, AWS, Snowflake, Alation, Collibra, Informatica, Tableau, BigQuery, Looker and more. What’s more, Apexon leverages pre-engineered accelerators to speed up data quality improvement.
- Maintain laser focus on objectives
A common pitfall in big data projects is that they become unwieldy, time-consuming, and slow to deliver ROI. This is often because enterprises try to do too much too soon and end up biting off more than they can chew. Mitigate against this by regularly checking that you’re keeping to your original objectives for delivering value while avoiding distractions from your scope.
- Track and resolve data quality issues fast
Even when your systems and tools are primed to spot quality issues early, with a rapidly evolving data landscape, quality issues can creep in. A robust digital assurance framework will increase agility across the whole data lifecycle, but it’s also worth educating employees to question data and flag quality issues. Seeing data quality be taken seriously encourages all those in the organization to be more accountable. Furthermore, understanding the root cause of a problem helps ensure it doesn’t happen again.
Data Quality as a Competitive Differentiator
Improving data quality enables companies to be more efficient, more agile, and make better decisions. And since a great many of the applications and technologies we associate with advancing digital maturity are data-intensive, ensuring the integrity of that data is fast becoming an essential component in any data governance strategy. As Gartner’s Melody Chien summarized, “Good quality data provides better leads, better understanding of customers, and better customer relationships. Data quality is a competitive advantage.”
For many organizations, the growth in volume and importance of data has left them struggling to control it. Putting that data to work so that businesses can glean actionable intelligence is among the primary reasons for undertaking digital transformation. However, even without a wider transformation program, enterprises can take steps to boost data quality and as a result, improve agility, decision-making, and performance.
If your enterprise is experiencing a big data challenge, Apexon’s team of data architects and digital engineers is equipped to alleviate your data pain-points. To find out how we can help resolve the toughest data challenges facing businesses today, check out our Data Services or get in touch using the form below.