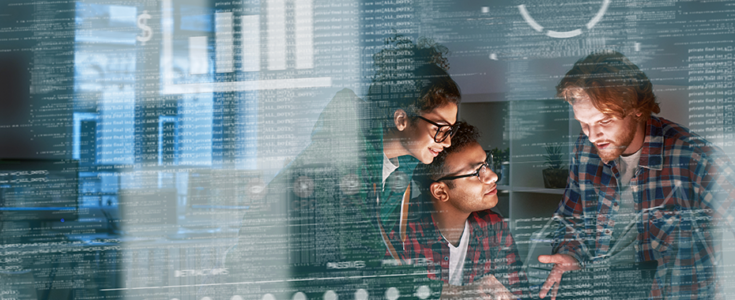
Everyone knows data is at the core of every business decision. If the data supporting the decision is high quality, it is usually a sound choice. The opposite is also true for bad data. According to Gartner, poor data quality data costs organizations $12.9 million yearly on average. It’s worth it to try to establish some control. But how? The answer: Data management.
Data management helps to ensure companies have quality data on which they can rely. Data management goes beyond simply controlling data by establishing a centralized governance and management model. This creates a streamlined, standardized system for organizations to effectively create, integrate, disseminate, and manage data for all enterprise applications, processes and entities requiring timely and accurate data delivery. There is no one-size-fits-all approach for data management. However, adhering to a set of proven data management best practices can provide a great foundation for your business intelligence.
Data Management Best Practices
One main area of concentration relating to best practices for effective data management is “Knowing your own Data,” meaning enterprises should invest in business/functional SMEs who understand and describe their business data sets. Enterprise should invest in building a business architecture to layout the functional building blocks for their business that then maps to associated business data domains, subject areas, and then to associated data assets.
To ensure data is consistently reliable, it’s important for businesses to implement best practices for data management.
1.) Prioritize high quality, standardized data
Business leaders wishing to stay ahead of the competition may want to explore making data quality a top priority within their organizations. For data to be effective, it needs to be clean and reliable. Using clean data in analytics/reporting increase accuracy and helps to fortify decision making. Inaccurate data can adversely affect departmental decision-making and the overall financial health of a company. By purging old data and checking regularly for accuracy, companies can help to ensure the quality of their data.
Data management is a team effort. Handled manually or automated, it’s essential to train team members on managing data and software. The efforts put into the collection and input of data are key for proper data management.
2.) Create a data recovery strategy (because accidents happen)
Accidents happen and with data, we know it’s not good when they do. Limited or no access to customer data can leave your company vulnerable to missed opportunities and revenue loss. Automation efforts, marketing campaigns, and sales strategies can be severely compromised due to data loss or inaccuracies. To avoid catastrophic business failure, a data recovery strategy is necessary.
Creating a data recovery strategy is the best way to safeguard against data loss. In order to generate data backups to restore systems in case of an accident, companies can choose to duplicate data centers, use non-critical servers, or move their operations to off-site locations. It’s important to have proper security permissions in place so that account access is always available.
3.) Consider metadata for data sets
Metadata enables and helps to improve use of data. An advantage is that good metadata can make up for data loss due to human error. Above all, metadata ensures data is FAIR. Meaning, data is findable, accessible, interoperable, and reusable.
4.) Consolidation of data into data warehouse, data vault, and data lakes
Data consolidation takes data from disparate sources, cleans it up, and combines it in a specific location. Based on individual needs, companies must decide between on-prem and cloud-based storage.
The following are some common misconceptions relating to storage:
5.) Data security
By definition, data security is the practice of protecting digital information from unauthorized access, corruption, or theft throughout its entire lifecycle. One of the biggest issues with data security is the degree of vulnerability faced by companies with older systems. Concerns directed at legacy systems include out-of-date security, the inability to apply additional layers of security, and the cost to maintain these systems.
To enhance security, organizations can migrate their data to newer systems that include data security features. Data protection measures can include access control and encryption. By granting specific privileges to certain users or turning data into meaningless code, it prevents abuse or use of data by unauthorized individuals.
6.) Data models
Data models are visual representations of an enterprise’s data elements and the connections between them. It includes creating a simplified diagram of a software system and the data elements it contains, using text and symbols to represent the data and how it flows. The goal of data modeling is to organize and standardize data, so it is easier to understand.
Here are a few common data models:
7.) Data governance
Processes for data collection and standardization help ensure that companies have access to critical data at the time it’s needed. Creating a data governance framework helps to increase productivity and manufacture an atmosphere where critical data is managed and protected.
Bonus: #8 Hire a data management service provider
Adhering to the 7 best practices above can help you to effectively manage your data on your own. However, prioritizing high quality data, implementing recovery strategies, consolidating and modeling data may be a difficult balancing act. Hiring a data management service provider can augment your internal resources. A DM expert often has greater exposure and experience in data management and a better understanding of industry trends and best practices. Based on their expertise, they can effectively tackle critical business challenges. In addition to experience, a service provider can also prove to be a cost-effective solution for your organization.
Apexon helps enterprises to succeed on their journeys to reach data maturity. If you’re interested in learning more about data management or hiring a service provider for your needs, check out Apexon’s Data Management and Governance services or get in touch with us directly using the form below.