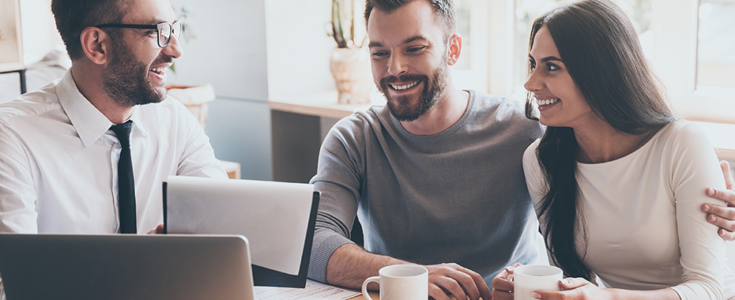
8 Use cases of AI/ML in the Mortgage Industry
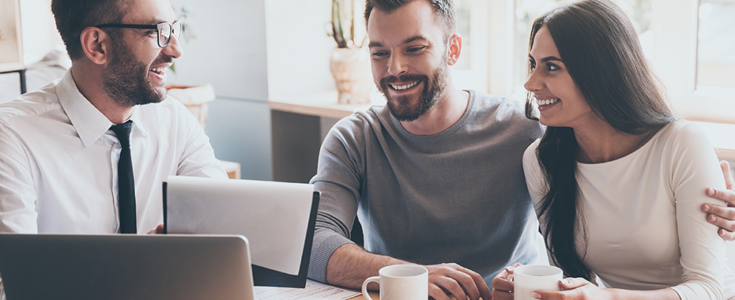
In the age of acceleration, artificial intelligence (AI) and machine learning (ML) are the key ingredients. AI enables the creation of new adaptive algorithms that are data sensitive and produce instant, automated, tailored, “smart” results. ML, a subset of AI, is potentially even more transformative, unleashing new possibilities for computers to learn and optimize without explicitly being programmed. Together, these technologies create a breakthrough that is transforming many industries.
The mortgage industry is one such sector being reshaped by advanced technology. The mortgage lending processes can be time-consuming, tedious, and frustrating for both parties. However, machine learning, artificial intelligence, and predictive analytics are changing this by streamlining workflows, reducing required human intervention, and automating critical decisions. In recent times, mortgages have started leveraging AI technology and ML algorithms for delivering smart solutions. With the integration of predictive analytics, human intervention will be reduced, and the decision-making will be automated.
Use Cases of Machine Learning in Mortgage Industry
1. Supervised Learning in the Mortgage Industry
Supervised learning is a machine learning technique that can be used to analyze and predict outcomes for applications in the mortgage industry. In a supervised learning model, a dataset of historical mortgage application data is used to train the model. The model is then used to predict outcomes for new mortgage applications based on the features of the applicant and the loan.
For example, a supervised learning model could be trained to predict the likelihood of a mortgage application being approved or denied based on the applicant’s credit score, income, and other factors. Additionally, the model can be used to predict the interest rate or the loan amount that will be offered to the applicant. Supervised learning can be very useful in automating the mortgage application process and making it more efficient.
2. Improved Application Verification
AI companies have the capacity to help with verification, which is beneficial for borrowers as well as lenders (the lenders can access historical data by training the predictive models). This helps with an accurate estimation of income levels, respective to potential factors. Moreover, automated assessment improves expense and income verification and helps outline the need for manual reviews in case of high-risk situations.
3. Better Document Validation
When it comes down to mortgage lending, it can be document intensive. The lenders must validate the information for consistency, completeness, accuracy, and correctness. In these cases, machine learning models can be optimized to validate the information. The ML models can outline and compare the text within the document which ensures that forms are filled out accurately with no errors. In addition, it allows the loan officers to focus on manual tasks that cannot be automated.
An area where machine learning can really change the game is in reviewing of the loan application for completeness, ensuring that the information entered in the application is consistent with the one available in the uploaded documents and fraud detection in general. This is completely feasible because technologies like natural language processing and neural networks are enabling machines to “read” the documents and recognize the pictures. Machines helping process the loan applications will not only minimize the processing time and make the process more effective but will also enhance the customer satisfaction in the process.
4. Methodology
The methodology associated with machine learning allows the automatic classification of mortgage-focused documents. These documents include bank statements, pay slips, valuation documents, legal documents, correspondence, and income assessments. It will ensure that information is readily available for smart decision-making while reducing costs and risks caused by human error.
5. Application Queue Prioritization
The mortgage lending process involves back and forth between the lender and the borrower. Loan officers must contact applicants frequently to communicate next steps and move the process forward. As lenders gather data on how consumers move through the process, respond to communications, and take actions, they can train machine learning models that optimize the loan officer’s workflow and recommend their next best action. This lets loan officers work through their queues with more speed and accuracy.
AI can be used to prioritize mortgage applications in a queue based on the likelihood of approval or other factors. A supervised learning model can be trained on historical mortgage application data to predict the likelihood of approval for new applications. The model can then be used to automatically prioritize applications with a higher likelihood of approval, allowing loan officers to focus on the most promising applications first. Additionally, the model can be used to identify applications that require additional review or documentation, and flag them for further review.
Another way to prioritize the applications is using reinforcement learning, where the agent learns to prioritize the applications based on the rewards or penalties it receives for its actions. For example, prioritizing an application that results in a successful loan closing will result in a positive reward, while prioritizing an application that is later denied will result in a negative penalty. Over time, the agent will learn to prioritize applications that are more likely to result in successful loan closings.
In both cases, using AI for mortgage application queue prioritization can help streamline the loan approval process and increase the efficiency and effectiveness of the loan officers.
6. Underwriting and Loan Pricing
Mortgage underwriting involves assessing the credit risk of an applicant and whether they meet the standards established by the lender and others. Traditional mortgage underwriting has been rules-based, where application information is run through a series of pass-or-fail rules that determine loan eligibility. Increasingly, model-based approaches are being used, wherein applications are scored by predictive models that determine eligibility. While some types of mortgages—such as those insured by the federal government—must follow specific rules and have limited underwriting flexibility, other mortgage products afford lenders more leeway. In these cases, lenders are increasingly looking to machine learning as an effective way to create predictive models for assessing credit risk.
For both underwriting and pricing processes, using ML can help to make the process more efficient by reducing the time and resources required for manual underwriting and pricing decisions. Additionally, ML models can be trained on a large amount of historical data, which can help to identify patterns and trends that may not be immediately obvious to loan officers. This leads to more accurate underwriting and pricing decisions.
7. Customer Retention
Machine Learning can predict the probability of customer retention and the risk of default by the customers. This information can then be effectively used by the mortgage companies to take proactive steps to prevent such scenarios from happening. They can offer loan modification programs or other alternatives to such at-risk customers.
8. AI/ML for Equal Opportunity Lending
Research has revealed decades-long race and gender bias in the mortgage lending industry. A study undertaken by Woodstock Institute in Chicago in 2015 found that women were 14.5% less likely to get loans compared to their male counterparts. Similarly, a study undertaken by National Community Reinvestment Coalition (NCRC) in Baltimore in 2015 found that white people were twice as likely to get loans even if they were not as qualified as the Black people who also applied.
People of color account for majority of the population growth in United States. Coupled with the population of women, this is a huge, missed business opportunity that can be served by mortgage lenders through machine learning algorithms. When machines provide the recommendations based on a set of objective criteria, decades of biases will start melting away and reveal new revenue streams for mortgage companies.
Features of a Well-Built ML Models for Mortgage Industry
- Model deployment – GUI-based interface to deploy models using REST APIs and simultaneously maintain metadata on deployed models
- Model management – Manage model versions across multiple deployments to ensure transparency and easy administration of multiple models
- Model performance – Monitor model performance of deployed models and get automated notifications for recalibration of models when performance drops below a defined threshold
- Explain ability – The ability for users to monitor and explain the rationale behind why the model is taking a specific decision
- Embedded into workspaces – Easily integrated with transactional systems and commonly used systems (CRM and payment systems)
- Role-based access – Advanced role-based access for model management
AI/ML and the Future of Mortgage Lending
Mortgage is a $18 trillion industry in United States. However traditionally, it has fallen behind the digital transformation curve. Hence, the sector is filled with untapped technological potential. Artificial intelligence and machine learning are powerful technologies that can not only delivery strategic growth to mortgage companies but also a superlative, smooth, and satisfying experience to the customer. With increased speed, efficiency, and accuracy and decreased costs, compliance woes, and prejudice, AI/ML will continue to have increased applications within the mortgage lending industry.
Apexon helps enterprises maximize the value of complex data ecosystems with its advanced analytics and AI/ML services. Complexity, poor data management, and ill-equipped infrastructure and tools are all obstacles to effectively putting your data to work for your business. If you’re interested in learning further about the power of modern analytics and data, check out Apexon’s Advance Analytics and AI/ML services or get in touch with us directly using the form below.