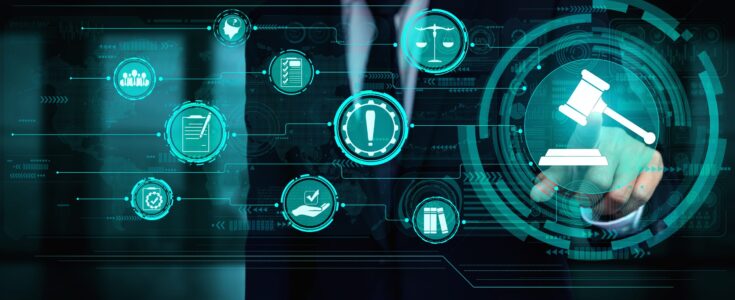
This article will talk about the need for maturing the QA process from Ad-hoc to optimization and how this can help enhance testing in the software development environments.
Contrary to popular belief, data governance and data management are two totally desperate practices. Data governance establishes data policies and procedures while data management puts them into action. Both data governance and data management are important action items for any organization dealing with large amounts of data as both can influence the quality of data and performance of an organization. For data stewards to truly make use of data effectively, it is essential to understand the difference between data governance and data management and how they align.
What’s Ahead
This article will help you understand the differences between data governance and management by answering the following key questions about them –
Data Governance
Data governance is a collection of defined structures, rules, policies, processes, owners, users, and measurements.
Policies and Rules: Policies and rules are created to address the concerns of quality, security, compliance, monitoring, and transparency. For example, while collecting data, a rule might state the need for getting consent from the creator. A policy would typically determine how data would be recorded, cleaned, documented, and categorized. It would typically include terms related to domains, accounts, systems, accounts, databases, and the data itself. The steps to assure these would require establishing management processes that are also defined in the data governance blueprint.
Roles and Responsibilities: To manage data processes, an organization would require data creators, owners, and users. Various roles are thus defined to give clarity on their rights and responsibilities. These roles could include:
The data governance framework also suggests creation of committees for data governance and management to separately manage the two disciplines.
Technical Metrics: Technical metrics are used to assess data quality and its impact on an organization. For example, duplicity, accuracy, completeness, and encryption could be the measured of data quality. For assessing organizational impacts of data processes, specific business metrices are often used.
While data governance appears to a be a defined set of practices to follow, these practices are not exclusive but intertwined. Thus, to ensure that data governance is successful in an organization, it must also follow a structure. Data governance is usually structured around certain principles such as integrity, transparency, stewardship, auditability, accountability, standardization, and change management. Some examples of these principles in action are:
Data Management
Data management is an IT practice used to provide timely access to data by managing the life cycle of data assets from creation to retirement. It meets data governance at the point where action begins to follow the guidelines identified in the governance framework. Technically, it involves implementation of rules, procedures, terms, and policies defined in data governance. Key activities involved in data management are:
The connection between data governance with data management clearly states that the benchmarks set in the governance frameworks must be taken care of while performing management activities. For instance, while performing any activities involved in data management such as preparing, extracting, transforming, and loading data:
The success of data governance is largely dependent on data management. Several data management tools and techniques are used to ensure that these activities are performed in accordance with the governance framework. Several principles of data governance and data management overlap such as accountability, integrity, compliance, and transparency. Here are a few examples of how their aligned principles are subscribed to in data management practices:
Data management practice adds more specific principles to the list such as protection, availability, retention, and disposition. For instance, to protect data, only authorized access is provided to genuine users of data while others are prevented from accessing the same. When data is moving out of the organization’s safe circle, encryption is used to protect it from reaching unauthentic users. Similarly, the data retention principle suggests that any data that is captured from users of a system must be maintained for a specific period after which it can be deleted. NSF Engineering Directorate suggests that any research data must be maintained for a period of three years after it is released publicly.
Data Governance vs Data Management
Data delivers insights. Insights support decisions. Decisions create growth. And when ideas meet data with this growth potential, innovation is produced.
It all starts with data and to extract insights from it, it needs to be organized and understood. For this, we use data management and data governance. They are often confused as one but are two very different concepts. Data management is a practice of organizing data while data governance provides a strategic framework to help make it possible. An organization needs to use both in tandem and in coordination with each other to get the most out of data. While governance would define procedures and policies, data management would act upon them.
Data governance success is the outcome of good data management while the latter is shaped by the governance structure. When they go together, success is achieved.
To take the next step in your data maturity journey, check out Apexon’s Data Governance and Data Management services or get in touch using the form below.