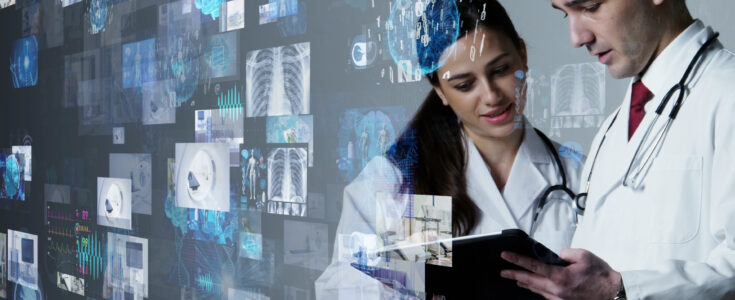
Harnessing AI/ML for Early Cancer Detection
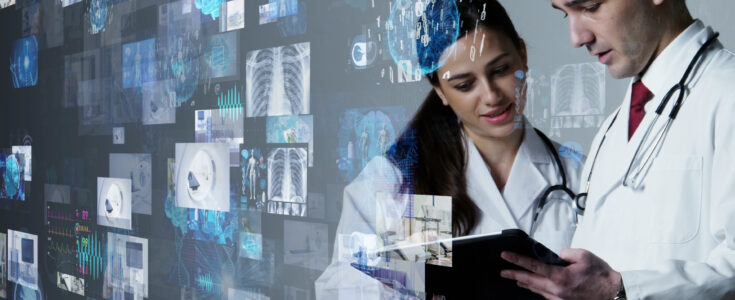
According to the World Health Organization, cancer accounts for approximately one in six deaths globally. Disease prevalence and progress is affected to an extent by factors we can control, like diet and lifestyle, but also by those we can’t, like genetics, or the country and health system into which you’re born. There’s a strong correlation between diagnosing patients early, health outlooks and survival rates. One of the areas where AI can be of greatest benefit is in the early detection and diagnosis of cancer.
When we talk about AI, it is really a shorthand way of referring to a wide range of data science disciplines, including ML, neural networking, cognitive computing, deep learning, computer vision, and more. This is because cancer testing and screening take many forms. Standard screening relies on imaging techniques to detect cancer and is used for a wide range of cancers, including the two most common cancers globally, lung and breast cancer. Screening is valuable, particularly when health services are able to detect and diagnose cancers early, but it’s not an easy job. Interpreting the images relies on highly skilled individuals. It’s a resource-intensive process, and it’s not perfect.
AI-assisted screening
Detecting cancer early, or even better, identifying cells at the pre-cancerous stage before they cause any symptoms, helps doctors treat cancer more effectively. There is always a concern around false positives, which can result in invasive and unnecessary procedures, and false negatives, in which cancerous growths are missed and patients are lulled into a false sense of security. That’s why the excitement is almost palpable when AI systems evidence equal or increased accuracy in detecting cancers. A recent Google Health and Imperial College London study revealed that AI was more accurate than doctors in diagnosing breast cancer. A joint initiative between Northwestern University and Google is improving the lung cancer detection and is now moving towards clinical adoption. Research like this hints tantalizingly at the potential of such technologies to be rolled out in a public health setting. Increased accuracy means better health outcomes as well as easing the AI the pressure on doctors as AI assists in the diagnostic process. As an additional benefit, AI systems do not need to rest as real doctors do.
Harnessing genomic data for better detection
Genetic predisposition is a major predictor of a person’s risk of cancer and we’re seeing significant advances in the research area where cancer detection, genomics and data science overlap. From a data science perspective alone, the challenges can be daunting. Data may be plentiful, but irregular in the way it’s been collected and in this case a data engineering program will be required as the foundational step before embarking on the development of the AI algorithm. By contrast, in some cases, there is simply not enough, in which the challenge is how to train a system without sufficient inputs. (Answer: training in reverse has been a successful strategy, utilizing synthetic data in the first instance to help the neural network learn until such time that there is enough “real” data.)
A further challenge when working with patient data is the clear need to protect patient privacy, meaning that data must be handled in accordance with the latest regulations, such as HIPAA. At Apexon, we have developed an accelerator, the Healthcare Data Analytics Platform (HDAP), that helps companies harness their healthcare data assets quickly, in compliance with the necessary regulations.
Accelerating diagnostics with data engineering
Apexon recently worked with a cancer diagnostic company which provides AI tools to pathologists to enable them to become faster and more accurate in diagnosing disease and recommending treatment for cancer patients. Our engagement included helping to accelerate the data engineering and data science aspects in order to train the neural network to be able to detect and diagnose cancer.
Refining and organizing the data inputs was a major part of developing the AI system to be able to identify cancer even in the early stages. This was done by “teaching” the algorithm what abnormal cell growth looks like for a wide variety of cancers, involving labelling a large number of pathology images, which were often low resolution and blurry. Image quality presented further challenges too since downsampling the images was necessary to feed them into the neural network, but reducing resolution could also result in the loss of crucial information. Cancer detection in imaging is just one part of the process. The non-Boolean nature of the diagnostic process – cognition, understanding of clinical context, perception, empirical experience – was another key skill to teach the AI system. Thanks to Apexon’s expertise in data engineering and site reliability engineering (SRE), the company’s diagnostic processes became more efficient.
Harnessing health data for better outcomes
Disparate data sources create widespread challenges in healthcare. Cancer detection using AI/ML is a prime example of the benefits of advance data analytics in healthcare, but the principles can be applied more widely in the health ecosystem. Complex, siloed systems and a lack of data standardization hinder important information access and negatively impact the quality of patient care, operational efficiency and revenue growth. Apexon enables entities across the healthcare ecosystem to leverage all their data assets to deliver higher service levels, increase operational efficiency and achieve financial performance.
Do you have a digital health challenge? Let us know using the form below.