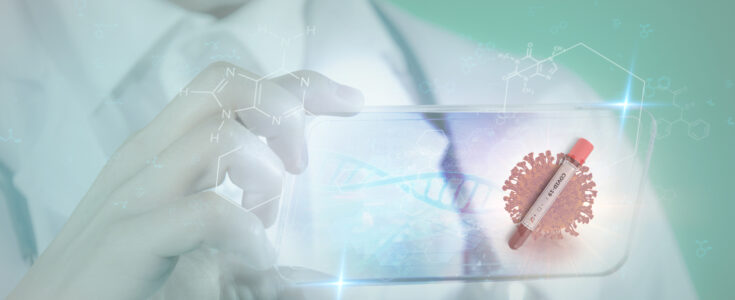
How Digital Twins Can Transform Patient Diagnosis & Treatment
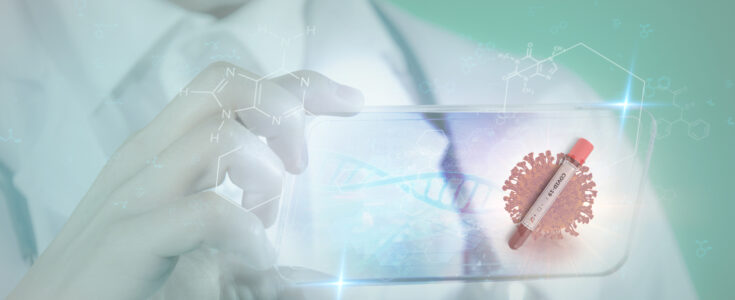
The connected society has been the catalyst for a plethora of digital initiatives and innovations, but the increased adoption of the internet of things across every industry is providing companies with numerous opportunities to digitalize processes that would otherwise have been physical. And while the shift from a reactive stance to a proactive attitude is arguably a work-in-progress, the utilization of next generation data and analytics technologies is certainly gathering pace.
Digital twins are a perfect example of this evolving awareness of what can be done in terms of leveraging actionable insights and delivering business outcomes.
The concept, which was introduced officially to an engineering conference in 2002, has already been applied to the manufacturing and automotive sectors, but its benefits are not limited to heavy machinery or connected industrial assets. In fact, the first practical application of the “digital twin” was demonstrated by NASA back in 2010.
On a very simple level, the digital twin is a virtual real-time representation of a physical asset or process. Based in the cloud, the digital version mimics the millions of data points that are generated by said assets or processes, allowing companies to see a living model of what is happening. When you are talking about assets that could cost millions of dollars to replace, then the insights that the twin provides are invaluable.
In recent years, the concept has moved from its engineering origins to encompass other industries, such as healthcare and the supply chain.
For the purposes of this blog post, it is the former that we want to highlight. To be more specific, we will be looking at how digital twins can enhance the quality of treatment for Chronic Obstructive Pulmonary Disease (COPD) and how they can be an effective tool for healthcare providers or doctors.
What is COPD?
According to the World Health Organization, COPD is the third leading cause of death worldwide, killing 3.23 million people (about the population of Arkansas) in 2019 alone. And while it is common, preventable and treatable, this lung disease is a global problem.
At its core, COPD affects the lungs and causes an individual to have difficulty breathing. When the disease takes hold, the lungs become inflamed and thicker than normal, which prevents the flow of oxygen.
When that happens, the tissue where oxygen is exchanged is damaged and the air flow in and out of the lungs decreases. Less oxygen is available for the body’s tissues and waste carbon dioxide (which is generated naturally) is retained. As the disease progresses, the shortness of breath that a person now experiences becomes a major problem – if you are interested in learning more about COPD, this factsheet from the American Lung Association is a good place to start.
It should be noted that COPD is not curable, but it is treatable. The key point to remember is that (like many other diseases) if left untreated, then it can cause serious health issues, with death the likely outcome. Treatment can take the form of antibiotics or steroids (depending on the severity of the condition), while there may be a requirement for inhalers or nebulized medicine.
The question that we need to consider is how digital assets or processes can alleviate or prevent the suffering that COPD causes. For that reason alone, the digital twin can be a lifeline for patients and providers.
Digital Twins can Benefit Patient Care
As we have noted above, the digital twin is a virtual or living representation of a physical object, and one that draws heavily on data. That makes it a good fit for the healthcare sector, even more so when you think about the amount of information that doctors or clinicians need to do their job effectively.
By integrating a digital twin into the treatment of a disease such as COPD, there is an opportunity to visualize both simple and complex medical responses to a patient condition without administering medication to the physical aspect – in this case, this would be the patient.
Let us look at the following scenario.
A patient suffering from COPD gets admitted to a healthcare provider for treatment. Before the patient gets seen by a doctor or nurse, patient data is entered as an electronic health record (EHR) into the system. Once that is done, the EHR will generate a digital twin by using both the historic data available and the diagnosis reports from the physician.
This means that the patient is now generating data points which can be studied and analyzed, providing an effective diagnosis for ongoing treatment. In many cases, this will involve the use of healthcare-centric sensors (in the form of wearables or an implantable), which can track the progress of COPD and align with the diagnosis required for health vitals.
A recent paper published by the National Institute for Healthcare Research takes a deeper dive into these use cases, which you can read here.
The Role of Sensors
Sensors are used to accurately know the state of the patient and to extract the health vitals from the patient’s body. For patients experiencing COPD, these sensors must be of a high enough precision to obtain not only quality results but also to avoid false triggers.
The graphic below shows where doctors and clinicians can use ECG sensors to determine a set of rules for assessing the level of COPD in a patient.
Source: litfl.com
These rules are commonly defined as follows:
- Rightward shift of the P wave axis with prominent P waves in the inferior leads and flattened or inverted P waves in leads I and aVL.
- Rightward shift of the QRS axis towards +90 degrees (vertical axis) or beyond (right axis deviation).
- Exaggerated atrial depolarization causing PR and ST segments that “sag” below the TP baseline.
- Low voltage QRS complexes, especially in the left precordial leads (V4-6).
- Clockwise rotation of the heart with delayed R/S transition point in the precordial leads +/- persistent S wave in V6. There may be complete absence of R waves in leads V1-3 (the “SV1-SV2-SV3” pattern).
In terms of the digital twin, the use of these sensors will both determine the current state of COPD and the effects that it is having on the patient’s heart rate. However, the ECG is not the only place where sensors give a fuller picture. In fact, the following sensors are also part of the process:
AQI Sensors
Air quality is a major factor in COPD, with continuous or frequent exposure to potentially harmful gases or particle matters playing a significant role in how the disease progresses.
Air quality sensors, therefore, can be a critical part of detecting the triggers or conditions that relate to COPD – a list of these detectable triggers can be found here.
Breath Strap Sensors
Breathing rate is another key factor that allows the healthcare provider to make a feasible correlation between the heart rate and the blood oxygen level. For instance, this type of sensors provide the thoracic respiration value.
Temperature Sensors
Body temperature is a basic vital sign, not only in terms of how the body is responding to treatment but also any adverse effects. A temperature sensor can be used to read the body’s heatmap precisely, allowing the doctor to determine the best response to the disease.
Spirometer
Air volume being produced by the lungs is also a vital parameter for analysis of COPD. A spirometer device which is coupled to the system can effectively read this volume at regular intervals, adding another strong data point to the overall COPD diagnosis.
How the Process can be Simplified
As you can appreciate, all these processes, medical devices and assessments must work together effectively to provide both the answers that doctors require and the results that the patient needs. This is where the digitalization of care becomes increasingly important, and the entire process can be simplified using AWS tools.
The graphic below shows how these tools can be integrated as part of the digital twin and, importantly, overall patient care:
Block diagram of AWS Integration
As you can see, the Med Nodes (MN) are powered by AWS FreeRTOS to collect the sensor data in real-time from the patient. In addition, the flexible nodes are customized based on sensor density and cluster type. The data collected from the MN is pushed to the Edge Device – the Med Hub (MH) – which runs the AWS Greengrass Core.
AWS Sagemaker is used to run the training to generate data models, which can be accessed for the analysis. This tool utilizes the data from the Amazon S3 training bucket to run the training process, and then generates the inference model. This inference model is pushed to the Greengrass core of the MH.
The MH collects data from the EHR and creates a specific environment for the digital twin. As a result of this action, data models will be made available in the Amazon S3 Trained model bucket for both COPD and the medicine response rules.
Next, the algorithm utilizes the prescription as the formula and correlates the data (for example, the health vitals of the patient collected in real-time from the MN and EHR module). Once this has been done, the analysis applies the formulae to the digital twin, creates different recovery curves based on the model, runs inferences and generates predictions.
Finally, the MH filters the predicted data based on the confidence score. If the data passes the filter rule, it will be pushed to the training bucket for incremental inference model updates. For the record, the incremental model may be trained using the Random Forest Algorithm.
In a real-world scenario, the treatment of the disease would require the right prescription at the right time. By using a digital twin, doctors can validate the situation precisely by comparing the virtual version of a patient to his/her physical condition or history. In this case, the data generated by the twin will determine if the medication works as expected.
Additionally, the system will smartly predict various results based on the effects of time intervals in the administration of food and/or medicine. From a wellness point of view, integrating a digital twin into the process helps to identify or detect very granular information results – a food allergy, for example. This also alleviates the chances of a tough situation becoming infinitely worse, thanks to the application of complex algorithms that are designed to deliver the required data.
Digital Twins, Physical Results
As healthcare continues to evolve and adapt to the requirements of the connected society, there will be a defined need for providers and clinicians to understand the role that digitally available data plays in wellness. Every patient is different, but the ability to detect or identify common patterns will impact how we deal with treatable conditions.
The administration of medications to patients will also benefit from increased use of virtual tools, with systems learning what can be prescribed for a certain condition and, importantly, what might not work for a particular person.
Digital twins will be an essential part of the process, even more so when you factor in the actionable (and proactive) insights that can be gained from their integration. And while the reaction curve of a particular medication could find commonality among patients, simply being able to learn from previous experiences will be invaluable.
Data is now a commodity, and the healthcare industry can increase both the quality and effectiveness of treatment provided by utilizing the information-centric tools that are available. Digital healthcare has become the norm in recent years, integrating virtual versions of medical devices and people who need treatment for a particular disease is just a logical path to take.
Click here to learn more about Apexon’s digital health expertise. Alternatively, you can find out how Apexon’s digital engineers can help you advance your toughest digital health challenges by using the form below.