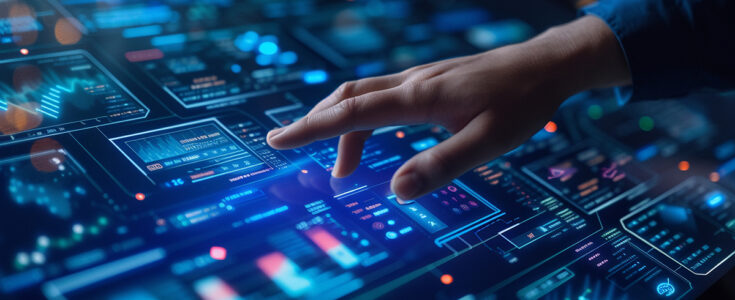
Strategic Technology Decisions in AI Deployment: Navigating the Path to Success
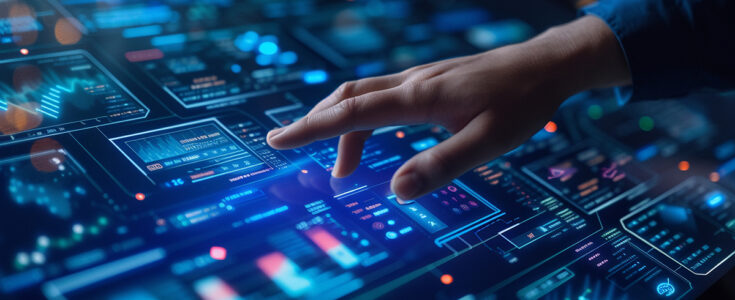
In today’s rapidly evolving digital landscape, the successful deployment of Artificial Intelligence (AI) solutions has become a critical priority for organizations aiming to stay competitive. However, deploying AI goes beyond technology; it requires a strategic blend of technical know-how, sound planning, and agility in addressing evolving needs. This article will explore key aspects of delivering AI solutions, technical considerations around open-source models, and high-level strategies that guide successful AI deployments.
Delivering AI Solutions: Key Considerations and Challenges
Delivering AI solutions isn’t as simple as flipping a switch; it involves a series of strategic decisions that revolve around the project’s objectives, infrastructure, and end users. The process requires thoughtful consideration of several key factors:
Also Read: Unleashing the Power of Azure AutoGen and AI Bots
1. Business Alignment
AI deployment must align with business goals and deliver value in a way that enhances decision-making, optimizes workflows, or improves customer experiences. The challenge lies in identifying the right use cases that can truly benefit from AI’s capabilities and integrating AI into the organization’s larger strategy.
2. Data Readiness
AI thrives on data, and the quality, quantity, and accessibility of data are crucial to building successful models. Poor data quality can lead to AI models producing irrelevant or even harmful results. Establishing a solid data pipeline, ensuring clean, consistent, and relevant data, and handling data governance and privacy considerations are critical.
3. Talent and Expertise
Skilled AI engineers, data scientists, and machine learning experts are essential for successfully developing, deploying, and managing AI solutions. However, there’s a talent shortage in AI, making it critical for organizations to invest in upskilling or hiring the right talent.
4. Model Deployment and Maintenance
Once AI models are developed, they must be deployed seamlessly into production environments. But it doesn’t end there—models require ongoing monitoring, retraining, and adjustments based on changes in the data or business environment. Ensuring that the models deliver consistent and accurate results is a key challenge.
Also Read: Keys to a Successful AI Strategy: Building for the Future
Tech Considerations and Scaling with Open-Source Models
Open-source AI models have democratized access to cutting-edge technology, allowing organizations to innovate without prohibitive licensing costs. However, while open-source models provide flexibility and a wealth of community-driven innovation, they also present their own set of technical considerations:
1. Customization and Adaptability
Open-source models are rarely plug-and-play. They often require significant customization to fit specific business needs. Teams must assess the model architecture, modify algorithms, and fine-tune performance. Open-source frameworks like TensorFlow, PyTorch, and Hugging Face offer flexibility, but using them effectively requires deep technical expertise.
2. Scalability Challenges
While open-source models are an excellent starting point, scaling these models to meet the needs of a growing enterprise can present significant challenges. From ensuring the model’s performance remains consistent under larger workloads to adapting to distributed computing environments, scaling requires strategic decisions about cloud infrastructure, edge computing, and computational resources.
3. Infrastructure and Tooling
AI models demand high-performance infrastructure for training, deployment, and monitoring. When working with open-source models, organizations must ensure they have the appropriate tools and platforms to support these activities. Leveraging cloud platforms like AWS, Azure, or Google Cloud, combined with tools like Kubernetes for container orchestration, can help organizations scale their open-source AI models effectively.
4. Ethical Considerations
Open-source models may be susceptible to biases or privacy issues embedded in the training data. Teams must integrate robust testing and bias detection methods into their AI workflows.
Case Study: US-Based Financial Services Firm Establishes GenAI CoE to Transform CX and Operations
An excellent example of successful AI deployment can be seen in Apexon’s collaboration with a US-based financial services firm. The organization sought to improve customer experience (CX) and operational efficiency through the establishment of a Generative AI Center of Excellence (CoE).
By leveraging Apexon’s expertise, the firm utilized Generative AI to streamline operations, automate routine tasks, and enhance customer interactions with predictive analytics. The implementation of open-source AI models and custom-built solutions helped the firm scale quickly and effectively. A key takeaway from this project was the importance of a strong AI strategy, cross-functional collaboration, and continuous refinement, which allowed the firm to transform its operations and significantly improve customer satisfaction.
Another compelling example of AI’s transformative power can be seen in Apexon’s partnership with a global clinical research organization. The organization faced challenges in extracting relevant patient burden information from unstructured clinical data. By utilizing Generative AI models, Apexon helped the organization automate the extraction process, reducing manual efforts and significantly improving data accuracy.
The GenAI solution allowed the organization to streamline patient data extraction, leading to faster and more accurate analysis of clinical trial information. This not only reduced the workload on the research teams but also ensured a more seamless experience for patients involved in clinical studies. This case highlights the potential of AI to revolutionize data-intensive sectors like healthcare by improving operational efficiency and enhancing decision-making processes.
Also Read: 3 Steps to Build an AI Foundation
High-Level Strategy for Successful AI Deployment
At the core of every successful AI deployment lies a high-level strategy that connects the technical and business aspects. Here are some guiding principles that help shape a successful AI strategy:
1. Clear Objectives and Roadmap
Without clear objectives, AI initiatives can quickly lose direction. Companies must identify which business problems AI will solve and outline a roadmap for implementation. Starting with smaller, well-defined use cases can provide quick wins and build momentum for larger AI initiatives.
2. Cross-Functional Collaboration
AI deployment is not the responsibility of one team; it requires collaboration across business units, IT, data science, and operations teams. Creating cross-functional teams ensures that AI initiatives are aligned with business goals, data is accessible, and the right infrastructure is in place.
3. Governance and Ethics
Establishing strong governance practices around AI is critical. This includes ensuring that models are compliant with data privacy regulations like GDPR, that they are free from bias, and that the decision-making process is transparent. Ethical AI use is not just a checkbox; it’s an ongoing commitment that requires robust policies and oversight.
4. Iterative Approach
AI deployment is an iterative process. As models are deployed, they must be monitored, evaluated, and refined. Organizations should adopt agile methodologies, allowing for continuous feedback and adjustment. Continuous monitoring ensures that models are responsive to changes in the environment, such as shifts in customer behavior or new market dynamics.
5. Talent Development and Upskilling
AI technology is evolving at a rapid pace. To stay ahead, organizations must continually invest in upskilling their workforce. Offering training programs and encouraging cross-functional knowledge sharing can help build a more AI-ready workforce, ensuring long-term success.
Conclusion
Strategic technology decisions in AI deployment can make or break a project. From understanding how to deliver AI solutions effectively, to making thoughtful choices about open-source models, and implementing high-level strategies that foster cross-functional collaboration and ethical AI use, success lies in careful planning and execution. As AI continues to evolve, organizations that invest in the right strategies and technology foundations will be better positioned to leverage AI for long-term business success.
Also read: Transforming Supply Chains with Generative AI – Part 1