Artificial intelligence (AI) is reshaping the banking, financial services, and insurance (BFSI) landscape at breakneck speed. From sharper fraud detection to smarter credit risk scoring and richer customer insights, the gains are undeniable. But with great power comes a hefty challenge: model risk. When bias creeps into AI models, it’s not just a tech glitch—it’s a leadership call-to-action. Here, we unpack where AI model risk comes from, why it’s a big deal for BFSI, and how to tackle it head-on, with Apexon as your go-to partner.
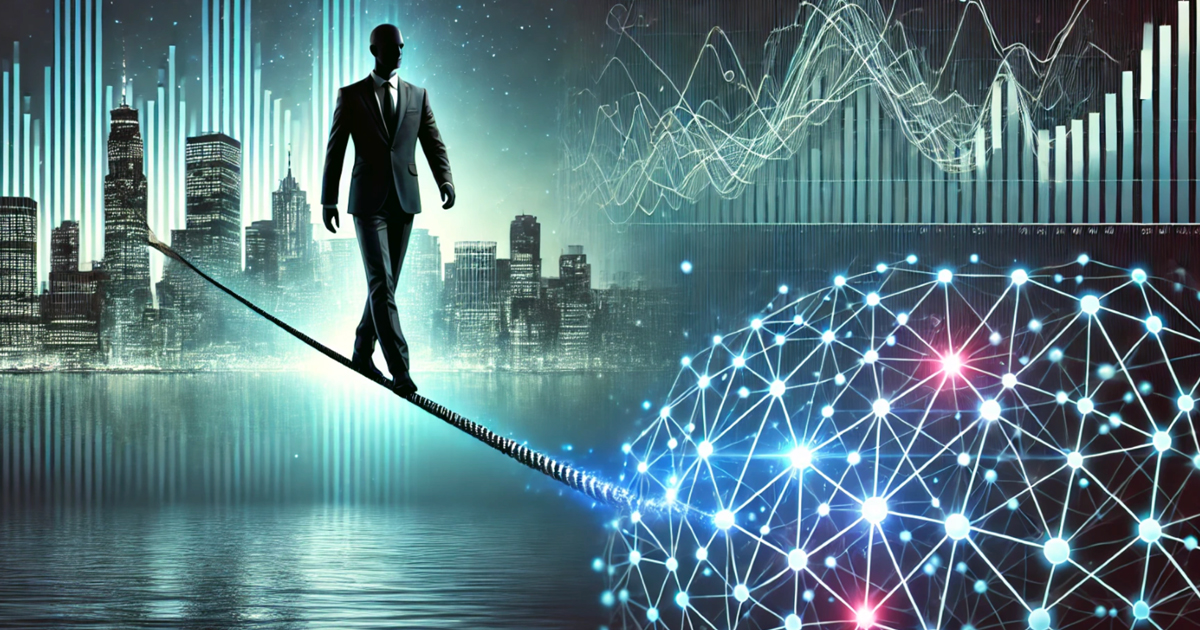
The Sneaky Saboteur: How Bias Wrecks AI Models
Bias in AI isn’t theoretical—it’s real and costly. Take Amazon’s hiring AI: it leaned toward male candidates by favoring resume buzzwords like “executed,” sidelining women. Or consider Carnegie Mellon’s finding that Google ads pushed high-paying gigs mostly to men. These aren’t outliers—they show how biased algorithms or skimpy data can churn out unfair, shaky results.
It’s not just about data, either. Context matters. AI leans on learned probabilities, ensemble dynamics, and neural network convolutions to predict outcomes. But throw it into unfamiliar territory—like a credit model trained on urban data judging rural borrowers—and it can flop. In BFSI, where trust and compliance are non-negotiable, these missteps hit hard, threatening both strategy and reputation.
Why BFSI Leaders Can’t Ignore This
AI model risk isn’t a sideline issue—it’s front and center. Look at recent headlines: the UK’s FCA probed AI credit scoring bias in 2023, while Wells Fargo took a $250M hit from the CFPB and OCC in 2022 over discriminatory lending tied to AI decisions. Regulators aren’t messing around. Post-2008, frameworks like the Fed’s SR Letter 11-7, the PRA’s Supervisory Statement, and the ECB’s TRIM have tightened the screws on model oversight. These aren’t optional checklists—they’re survival tools in an AI-driven world.
AI’s upside in BFSI is massive—quicker loan approvals, sharper fraud catches, deeper customer insights. But speed amplifies slip-ups. A spreadsheet typo is fixable; an AI model quietly favoring one group over another can tank compliance and trust. Regulators push fairness because customers demand it—whether it’s a mortgage or an insurance claim, people expect a level playing field. When bias skews AI, it’s not just a regulatory ding; it’s a betrayal of the trust BFSI runs on.
Three Steps to Tame AI Model Risk
Mitigating model risk isn’t rocket science—it’s disciplined execution. Here’s how to keep our AI systems solid, fair, and compliant.
1. Nail Data Quality
AI thrives on data that’s big and broad, reflecting real-world messiness. Diverse datasets beat bias early—think regularization (Elastic Net often trumps Lasso or Ridge) or data augmentation to fill gaps. Skimp here, and you’re toast.
2. Build and Test Like Crazy
Train rigorously—tune those hyperparameters, stress-test, backtest. Ask: How does this model hold up if rates spike or markets tank? Don’t forget cognitive bias from the team—those baked-in assumptions need airing out. It’s a grind, but it’s the safety net.
3. Bring in Fresh Eyes
Independent reviews spot what insiders miss. Cross-validation, paired with tools like Hugging Face’s metrics, keeps you honest. Some balk at the pace hit—too bad. In BFSI, one wrong move can spark a firestorm; thoroughness isn’t optional.
Apexon: Your Wingman in the Model Risk Fight
At Apexon, we get the BFSI model risk maze—and we’re already in the trenches with clients. Our data analytics and automation chops deliver solutions that nail compliance, fairness, and efficiency. Check our RegTech page or BFSI solutions to see how we roll.
Our track record speaks:
- Fraud Buster: Anomaly detection that flags oddities in real time, keeping risk at bay.
- Churn Predictor: An XGB Classifier on subscriber data helped a firm spot attrition early and act fast.
- Platform Overhaul: AI deployments streamlined ops for a big bank.
- Automation Win: End-to-end coding, workflow, and audit integration boosted accuracy and security for another client.
We start with data that works—curating sets that match reality. For one insurer, we fixed a claims model skewed by urban-rural gaps, cutting bias and boosting precision. Our stress tests simulate crashes and regulatory curveballs, keeping models tough. Independent validation aligns with SR 11-7 or TRIM—like when we retooled a bank’s lending AI, lifting approvals 12% in underserved markets without breaking rules. Dig into our data analytics services for more.
Apexon’s here with practical, people-smart fixes that don’t skimp on innovation. Hit us up to tackle your model risk challenges and score sustainable wins.
Industry’s inflection: Steer AI Right
By December 2025, AI in BFSI will be deeper—think real-time risk scores and hyper-tailored customer experiences. But that future rests on nailing model risk now. For us in the tech industry, this is our shot to juggle speed, scale, and fairness.
Step up: Scrutinize the data, push our teams to test every angle, and lean on partners like Apexon to plug gaps and meet regs like SR 11-7 or TRIM. We’re ready to help you build AI that’s cutting-edge and trustworthy. Connect with us to shape your strategy and secure a smarter, safer future.