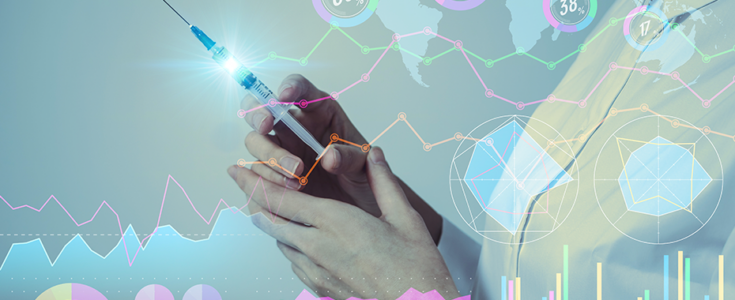
The complete and timely dissemination of clinical trial data is essential to all fields of medicine. Any delays or incomplete data release could have harmful effects on both patient care and scientific inquiry. Efficient use of data and AI techniques can have a substantial impact in the reporting of clinical study results and reduction in delays.
The Problem with Traditional Clinical Trials
Clinical trial delays have become the norm as 8 out of 10 trials will face delays. What’s more is 94% of delays are longer than one month. Scientists realistically spend about 10-20% of their time on problem solving or value creation tasks and the rest of their time on administration or manual transactions such as cleaning, processing, reviewing, and filing data. Further, the cost of bringing one drug to market is about $2.5 billion and only 10% of all drug candidates make it beyond Phase 1 of clinical trials. Therefore, the massive investment has a 90% failure rate!
Hence, it is paramount to reinvent the traditional data processing model in pharma that underpins current efforts in digital transformation. By acting on its desire to digitally transform, the pharma/clinical research organizations (CRO) industry has started adopting solutions such as automation to move away from manual processes and reducing some delays.
Applying AI in Clinical Trial Settings
By enabling digital and data-driven clinical trials with a pervasive AI/machine learning (ML) to automate manual processes within clinical trial settings, insights can be delivered faster and delays can be reduced. The areas of focus include:
AI is designed to deliver transformational value and accelerate complex decision-making throughout the clinical trial lifecycle. The advanced AI and ML capabilities add to the depth and breadth of the data-driven products within the data platforms, which can be the foundation of digital clinical trials. By taking a platform approach, pharma/CRO’s can:
The significant increase in data volume and variety has led to greater trial complexity. Even with the most comprehensive data review plans, issues can be missed. This can lead to costly and time-consuming errors. Seamlessly integrated with workflows in data platforms, AI-enabled data review capabilities for data managers and reviewers ensures data integrity in a more efficient, scalable way.
Leverage ML for study agnostic and subject-based multi-variate outlier detection to identify and mark exceptions across multiple data sources. Subject level data anomaly detection models can be applied to detect anomalies for safety domains (ex: vital signs, adverse events, etc.).
AI-enabled query/search automation capabilities are designed to predict and proactively manage data issues that are both operational and context-driven, simplifying query management processes and reducing manual burden for data managers and reviewers.
For outliers and anomalies detected by the system, ML and natural language generation (NLG) models are applied to auto-generate queries and recommend review actions to resolve queries raised. Over time, AI and ML models learn from historical data and data reviewer actions performed across trials to auto-resolve the generated queries and fix data issues.
Advancing Clinical Trials with AI
With AI-enabled data platform and review capabilities, the pharma/CRO companies can automate data pipelines, processing, review actions, error evaluation, and raising queries. This improves data quality, increases the speed of review, and reduces manual efforts at scale, thereby significantly reducing delays.
Apexon leverages the latest data techniques to accelerate digital initiatives and maximize business outcomes. To learn more about applying AI to your clinical trial scenarios, get in touch with our advanced analytics and AI/ML experts today using the form below.
This blog was cowritten by Dr. Ramyaa Ganesh, Mahesh Naphade, and Dr.Atif Farid Mohammad